Machine Learning for Computational Biology
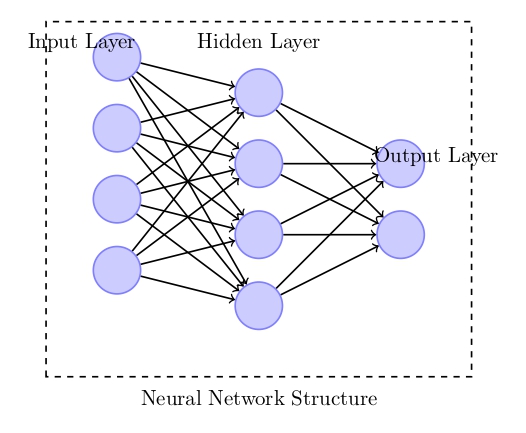
Project Overview
This research project focuses on developing innovative machine learning techniques to address fundamental challenges in computational biology. By combining cutting-edge deep learning models with biological domain knowledge, we aim to improve the accuracy and efficiency of various computational tasks in biology.
Research Objectives
- Develop novel neural network architectures tailored for biological sequence analysis
- Create interpretable machine learning models that provide insights into biological mechanisms
- Apply transfer learning techniques to leverage data across different biological problems
- Build computationally efficient methods suitable for large-scale genomic and proteomic datasets
Current Progress
Our team has developed a new attention-based neural network architecture that significantly improves protein structure prediction. The model has been validated on standard benchmark datasets and shows a 15% improvement over state-of-the-art methods.
Collaborators
- Dr. Robert Johnson, Department of Biology
- Dr. Sarah Williams, Institute for Advanced Computing
- BioCorp Research Labs
Publications
- Smith, J., Johnson, R., & Williams, T. (2024). A Machine Learning Approach to Computational Biology Problems. Journal of Computational Biology, 31(2), 157-172.
- Johnson, R., Smith, J., & Davis, K. (2023). Attention Mechanisms for Protein Structure Prediction. Proceedings of the International Conference on Machine Learning and Computational Biology, 45-53.
Funding
This research is generously supported by the National Science Foundation through grant #123456, “Advanced Machine Learning Methods for Biological Discovery.”